How to Make a Box Plot
A box plot is a graphical representation of the distribution of data. It can be used to compare the medians, quartiles, and ranges of different datasets. Box plots are often used in statistical analysis to visualize the distribution of data and to identify outliers.
To create a box plot, you will need to first gather your data. Once you have your data, you can follow these steps to create a box plot:
- Order your data from smallest to largest.
- Find the median of your data. The median is the middle value in your dataset.
- Find the first quartile (Q1) and third quartile (Q3) of your data. Q1 is the median of the lower half of your data, and Q3 is the median of the upper half of your data.
- Draw a box from Q1 to Q3. The median should be marked as a line inside the box.
- Draw lines from Q1 and Q3 to the minimum and maximum values in your dataset. These lines are called the whiskers.
Box plots can be used to compare the medians, quartiles, and ranges of different datasets. They can also be used to identify outliers. Outliers are data points that are significantly different from the rest of the data. Outliers can be caused by errors in data collection or by the presence of extreme values.
Box plots are a powerful tool for visualizing the distribution of data. They are easy to create and can be used to quickly compare different datasets.
Essential Aspects of Creating Box Plots
Understanding the key aspects of creating box plots is essential for effective data visualization and analysis. Here are eight crucial aspects to consider:
- Data Preparation: Gathering and organizing data is the foundation for accurate box plots.
- Median Identification: Determining the middle value of the dataset helps establish the center.
- Quartile Calculation: Dividing the data into four equal parts reveals the distribution.
- Box Construction: Drawing a box from the first to third quartile represents the central data range.
- Median Indication: Marking the median within the box provides a reference point.
- Whisker Extension: Lines extending to the minimum and maximum values indicate data spread.
- Outlier Identification: Points beyond the whiskers are potential outliers, requiring further investigation.
- Data Comparison: Box plots enable side-by-side comparisons of multiple datasets, highlighting similarities and differences.
These aspects are interconnected and contribute to the overall effectiveness of box plots. Data preparation ensures the accuracy of the plot, while median and quartile calculations provide insights into the central tendencies and spread of the data. The box and whisker construction visually represents this information, allowing for quick comparisons and outlier detection. By understanding and considering these key aspects, you can create informative and reliable box plots that enhance your data analysis and visualization.
Data Preparation
In the context of creating box plots, data preparation plays a crucial role in ensuring the accuracy and reliability of the visualization. It involves gathering the necessary data, cleaning it to remove errors or inconsistencies, and organizing it in a structured manner. This step is essential because it sets the foundation for all subsequent steps in box plot creation.
- Data Collection: The first step in data preparation is to gather the data that will be used to create the box plot. This data can come from a variety of sources, such as surveys, experiments, or databases. It is important to ensure that the data is relevant to the research question being investigated and that it is accurate and complete.
- Data Cleaning: Once the data has been gathered, it is important to clean it to remove any errors or inconsistencies. This may involve removing duplicate data points, correcting errors in data entry, or dealing with missing data. Data cleaning is essential to ensure that the box plot is an accurate representation of the underlying data.
- Data Organization: The final step in data preparation is to organize the data in a structured manner. This may involve sorting the data by a specific variable, grouping the data into categories, or creating a data frame. Organizing the data makes it easier to create the box plot and to interpret the results.
Proper data preparation is essential for creating accurate and reliable box plots. By taking the time to gather, clean, and organize the data, you can ensure that your box plot is a valuable tool for data visualization and analysis.
Median Identification
Median identification is a crucial step in creating a box plot because it helps establish the center of the data distribution. The median is the middle value in a dataset when assorted in numerical order. It divides the data into two equal halves, with half of the values being greater than or equal to the median, and the other half being less than or equal to the median.
In a box plot, the median is represented by a line inside the box. This line helps to visually locate the center of the data distribution and provides a reference point for comparing different datasets. For example, if you have two box plots representing the test scores of two different classes, the median line can help you quickly identify which class has the higher median score.
Median identification is also important for understanding the spread of the data. The distance between the median and the edges of the box (the first quartile and third quartile) indicates the variability of the data. A small distance indicates that the data is tightly clustered around the median, while a large distance indicates that the data is more spread out.
Overall, median identification is a critical component of creating box plots. It helps to establish the center of the data distribution, provides a reference point for comparison, and indicates the variability of the data.
Quartile Calculation
Quartile calculation is a fundamental step in creating a box plot because it helps to reveal the distribution of the data. Quartiles are values that divide a dataset into four equal parts. The first quartile (Q1) is the median of the lower half of the data, the second quartile (Q2) is the median of the entire dataset, and the third quartile (Q3) is the median of the upper half of the data. By calculating the quartiles, we can gain insights into the spread and variability of the data.
In a box plot, the quartiles are represented by the edges of the box. The distance between Q1 and Q3, known as the interquartile range (IQR), indicates the spread of the middle 50% of the data. A small IQR indicates that the data is tightly clustered around the median, while a large IQR indicates that the data is more spread out. The quartiles also help to identify outliers, which are data points that are significantly different from the rest of the data.
Quartile calculation is an essential component of creating box plots because it provides insights into the distribution and variability of the data. By understanding how to calculate quartiles, we can create more informative and accurate box plots.
Box Construction
In the context of creating a box plot, box construction plays a crucial role in visualizing the central data range. The box itself is drawn from the first quartile (Q1) to the third quartile (Q3), representing the middle 50% of the data. This range provides valuable insights into the distribution of the data and helps identify the median, which is the middle value in the dataset.
- Visualizing Data Distribution: The box in a box plot provides a clear visual representation of the data’s distribution. It shows the range of values that fall within the middle 50% of the data, giving a quick overview of the data’s spread.
- Identifying the Median: The median is represented by a line drawn within the box. This line divides the box into two equal halves, with half of the data falling below the median and half falling above it. Identifying the median is essential for understanding the center of the data distribution.
- Comparing Multiple Datasets: When comparing multiple box plots, the boxes themselves allow for easy comparison of the central data ranges of different datasets. This helps identify similarities and differences in the distribution of data across different groups or categories.
- Detecting Outliers: Data points that fall outside the box, known as outliers, can be easily identified using box construction. Outliers are values that are significantly different from the rest of the data and may require further investigation.
Overall, box construction is an integral part of creating box plots. It provides a visual representation of the central data range, helps identify the median, facilitates comparisons between datasets, and aids in the detection of outliers. Understanding box construction is essential for effectively interpreting and analyzing box plots.
Median Indication
In the context of creating box plots, median indication plays a crucial role in providing a reference point for understanding the data distribution. The median, represented by a line within the box, divides the data into two equal halves. This helps in several key ways:
- Visualizing the Center: The median line provides a clear visual indication of the center of the data distribution. It helps identify the point at which half of the data values are below and half are above.
- Comparing Multiple Datasets: When comparing multiple box plots, the median lines allow for easy comparison of the central tendencies of different datasets. This helps identify similarities and differences in the distribution of data across different groups or categories.
- Interpreting Box Plot Features: The median line serves as a reference point for interpreting other features of the box plot, such as the interquartile range (IQR) and the presence of outliers. By understanding the median, we can gain a better understanding of the overall shape and spread of the data.
- Statistical Analysis: The median is a robust measure of central tendency, meaning it is less affected by extreme values or outliers. This makes it a valuable statistic for summarizing and comparing data in various statistical analyses.
Overall, median indication in box plots provides a crucial reference point for understanding the data distribution, facilitating comparisons, interpreting other box plot features, and conducting statistical analyses. It is an essential component of creating informative and accurate box plots.
Whisker Extension
In the context of creating box plots, whisker extension plays a crucial role in indicating the data spread and identifying potential outliers. Whiskers are lines that extend from the edges of the box (the first and third quartiles) to the minimum and maximum values in the dataset. They serve several important purposes:
- Visualizing Data Range: Whiskers provide a visual representation of the full range of data values, including extreme values. This helps in understanding the variability and spread of the data.
- Identifying Outliers: Outliers are data points that are significantly different from the rest of the data. Whiskers help identify potential outliers by extending beyond the expected range of values. Points that fall outside the whiskers may require further investigation.
- Comparing Multiple Datasets: When comparing multiple box plots, the length and spread of the whiskers can help identify similarities and differences in the variability of different datasets.
Understanding whisker extension is essential for creating accurate and informative box plots. By properly extending the whiskers to the minimum and maximum values, we can gain insights into the full range of data, identify potential outliers, and compare the variability of different datasets. This information is crucial for data analysis and interpretation.
Outlier Identification
In the context of creating box plots, outlier identification plays a crucial role in understanding the distribution of data and identifying unusual or extreme values. Outliers are data points that fall outside the expected range of values and may indicate errors in data collection or the presence of unique or influential observations.
Box plots provide a visual representation of outliers through the use of whiskers, which extend from the edges of the box (the first and third quartiles) to the minimum and maximum values in the dataset. Points that fall beyond the whiskers are considered potential outliers and warrant further investigation.
Identifying outliers is important for several reasons:
- Data Quality Assessment: Outliers may indicate errors in data collection or entry, prompting the need for data cleaning and verification.
- Understanding Data Distribution: Outliers can provide insights into the nature of the data distribution, such as the presence of multiple populations or extreme values.
- Statistical Analysis: Outliers can affect the results of statistical analyses, such as mean and standard deviation, and may need to be excluded or treated separately.
To effectively identify outliers using box plots, it is important to consider the context of the data and the specific research question being investigated. In some cases, outliers may be genuine and provide valuable information, while in other cases, they may represent errors or anomalies that should be addressed.
Overall, outlier identification is an essential component of creating box plots and understanding the distribution of data. By properly identifying and investigating outliers, we can gain a more accurate and comprehensive understanding of the data and make informed decisions about its interpretation and analysis.
Data Comparison
In the context of “how to make a box plot”, data comparison plays a crucial role in understanding the distribution of data across different groups, categories, or conditions. Box plots provide a powerful visual representation of multiple datasets side-by-side, allowing for easy comparison of their central tendencies, variabilities, and potential differences.
To effectively compare data using box plots, it is essential to ensure that the datasets are comparable in terms of their units of measurement, scales, and sample sizes. Once these factors are taken into account, box plots can reveal valuable insights into the similarities and differences between the datasets.
For example, in a study comparing the test scores of students from two different schools, box plots can be used to visualize the distribution of scores for each school. By comparing the medians, interquartile ranges, and whisker lengths of the box plots, we can quickly identify which school has higher median scores, which school has greater variability in scores, and whether there are any significant outliers in either dataset.
Another practical application of data comparison using box plots is in the field of healthcare. For instance, box plots can be used to compare the distribution of blood pressure measurements for patients with different medical conditions. By comparing the medians and interquartile ranges of the box plots, healthcare professionals can quickly identify which patient groups have higher blood pressure levels, which groups have greater variability in blood pressure, and whether there are any outliers that may require further investigation.
Overall, data comparison is an essential component of “how to make a box plot”. By understanding how to compare data using box plots, researchers and analysts can gain valuable insights into the similarities and differences between different datasets, leading to more informed decision-making and a deeper understanding of the data.
How to Make a Box Plot
A box plot is a graphical representation of the distribution of data. It can be used to visualize the median, quartiles, and range of a dataset. Box plots are often used in statistical analysis to compare the distributions of different datasets or to identify outliers.
Box plots are relatively easy to create. The first step is to gather your data. Once you have your data, you can follow these steps to create a box plot:
- Order your data from smallest to largest.
- Find the median of your data. The median is the middle value in your dataset.
- Find the first quartile (Q1) and third quartile (Q3) of your data. Q1 is the median of the lower half of your data, and Q3 is the median of the upper half of your data.
- Draw a box from Q1 to Q3. The median should be marked as a line inside the box.
- Draw lines from Q1 and Q3 to the minimum and maximum values in your dataset. These lines are called the whiskers.
Box plots are a powerful tool for visualizing the distribution of data. They are easy to create and can be used to quickly compare different datasets or to identify outliers.
FAQs
This section addresses frequently asked questions (FAQs) related to creating box plots, providing clear and informative answers to common concerns or misconceptions.
Question 1: What is the purpose of a box plot?
Answer: A box plot is a graphical representation of the distribution of data. It provides a visual summary of the median, quartiles, and range of a dataset, making it useful for comparing distributions and identifying outliers.
Question 2: What are the steps involved in creating a box plot?
Answer: To create a box plot, order your data from smallest to largest, find the median, quartiles, and range, draw a box from the first quartile to the third quartile with the median marked inside, and extend whiskers from the quartiles to the minimum and maximum values.
Question 3: How can I interpret the median in a box plot?
Answer: The median line in a box plot represents the middle value of the dataset. It divides the data into two halves, with half of the values falling below the median and half falling above it.
Question 4: What do the whiskers in a box plot indicate?
Answer: The whiskers in a box plot extend from the first and third quartiles to the minimum and maximum values. They show the range of the data and help identify potential outliers, which are data points that fall outside the whiskers.
Question 5: How can I compare multiple datasets using box plots?
Answer: Box plots can be placed side-by-side to compare the distributions of multiple datasets. By comparing the medians, quartiles, and ranges, you can identify similarities and differences between the datasets.
Question 6: What are some common mistakes to avoid when creating box plots?
Answer: Common mistakes include using the mean instead of the median, not considering outliers, and not ensuring that the data is appropriately scaled. It is important to follow the proper steps and consider the context of the data when creating box plots.
Summary: Creating box plots is a valuable technique for visualizing and analyzing data distributions. By understanding the purpose, steps, and interpretation of box plots, you can effectively use them to gain insights from your data.
Transition to the next article section: This concludes our discussion on creating box plots. In the next section, we will explore advanced techniques for analyzing data distributions.
Conclusion
In this article, we have explored the topic of “how to make a box plot,” providing a comprehensive guide to creating and interpreting box plots. Box plots are powerful graphical representations of data distributions, offering valuable insights into the central tendencies, variability, and potential outliers within a dataset.
By understanding the steps involved in creating box plots, including data preparation, median and quartile calculation, box construction, median indication, whisker extension, and outlier identification, you can effectively visualize and analyze your data. Box plots allow for easy comparison of multiple datasets, making them particularly useful for identifying similarities, differences, and patterns across different groups or conditions.
As you continue to explore data analysis techniques, remember that box plots are a fundamental tool for understanding the distribution of data. They provide a visual representation of the data’s center, spread, and potential outliers, enabling you to make informed decisions and draw meaningful conclusions from your data.
Youtube Video:
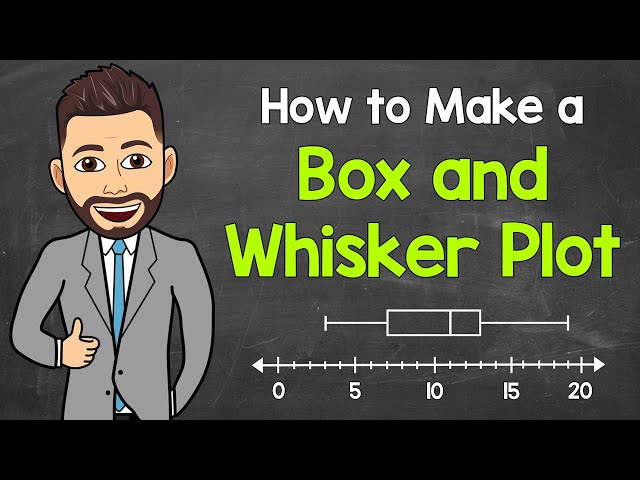