Displaying Authors in Data Science Projects
When working on a data science project, it is important to give credit to the authors of the data and code that you use. This can be done by displaying the authors’ names and affiliations in the project documentation, or by including a reference list at the end of the project.
There are several different ways to display authors in a data science project. One common approach is to create a table that lists the authors’ names, affiliations, and contributions to the project. Another approach is to use a visualization to display the authors’ names and affiliations. For example, you could create a network graph that shows the connections between the authors.
No matter which approach you choose, it is important to make sure that the authors’ names and affiliations are displayed prominently in the project. This will help to ensure that the authors receive the credit they deserve for their work.
Here are some benefits of displaying authors in data science projects:
- It gives credit to the authors of the data and code that you use.
- It helps to build a community of data scientists.
- It can help you to get feedback on your work.
- It can help you to find collaborators for future projects.
In conclusion, displaying authors in data science projects is important for giving credit to the authors of the data and code that you use, building a community of data scientists, getting feedback on your work, and finding collaborators for future projects.
Exploring the Key Aspects of Displaying Authors in Data Science Projects
When working on a data science project, it is important to give credit to the authors of the data and code that you use. This can be done by displaying the authors’ names and affiliations in the project documentation, or by including a reference list at the end of the project.
- Accuracy: Ensure that the authors’ names and affiliations are displayed correctly.
- Completeness: Include all of the authors who contributed to the project.
- Clarity: Make sure that the authors’ names and affiliations are displayed in a clear and concise manner.
- Consistency: Use the same format for displaying the authors’ names and affiliations throughout the project.
- Prominence: Display the authors’ names and affiliations prominently in the project.
- Transparency: Be transparent about the process used to identify and display the authors.
- Timeliness: Display the authors’ names and affiliations as soon as possible after the project is completed.
- Sustainability: Make sure that the authors’ names and affiliations are displayed in a way that is sustainable over time.
These key aspects are important for ensuring that the authors of data science projects receive the credit they deserve for their work. By following these guidelines, you can help to build a community of data scientists and encourage collaboration.
Accuracy
Accuracy is important in displaying authors in data science projects because it ensures that the authors receive the credit they deserve for their work. Incorrectly displaying authors’ names or affiliations can lead to confusion and can make it difficult for others to find and cite the work.
- Consistency: One aspect of accuracy is consistency. It is important to use the same format for displaying authors’ names and affiliations throughout the project. This will help to ensure that the information is clear and easy to understand.
- Completeness: Another aspect of accuracy is completeness. It is important to include all of the authors who contributed to the project. This includes authors of data, code, and other resources.
- Transparency: Accuracy also requires transparency. It is important to be transparent about the process used to identify and display the authors. This will help to build trust and ensure that the information is accurate.
By following these guidelines, you can help to ensure that the authors of data science projects receive the credit they deserve for their work. Accurate and complete author information is essential for building a community of data scientists and encouraging collaboration.
Completeness is an important aspect of displaying authors in data science projects because it ensures that all of the authors who contributed to the project receive credit for their work. Incomplete author information can lead to confusion and can make it difficult for others to find and cite the work.
For example, if a data science project is based on data from multiple sources, it is important to include the authors of all of the data sources in the project documentation. Similarly, if a data science project uses code from multiple sources, it is important to include the authors of all of the code sources in the project documentation.
Including all of the authors who contributed to a data science project is also important for building a community of data scientists. When authors are properly credited for their work, they are more likely to share their work with others and collaborate on future projects.
In conclusion, completeness is an essential aspect of displaying authors in data science projects. By including all of the authors who contributed to the project, you can help to ensure that they receive credit for their work, build a community of data scientists, and encourage collaboration.
Clarity
Clarity is important in displaying authors in data science projects because it ensures that the information is easy to understand and find. Unclear or concise author information can lead to confusion and can make it difficult for others to find and cite the work.
For example, if the authors’ names are displayed in a long and complex format, it can be difficult for readers to quickly identify the authors. Similarly, if the authors’ affiliations are not displayed in a clear and concise manner, it can be difficult for readers to determine the authors’ institutional affiliations.
Clarity is also important for building a community of data scientists. When authors are clearly and concisely identified, it is easier for others to find and collaborate with them. This can lead to new collaborations and new data science projects.
In conclusion, clarity is an essential component of displaying authors in data science projects. By ensuring that the authors’ names and affiliations are displayed in a clear and concise manner, you can help to ensure that the authors receive credit for their work, build a community of data scientists, and encourage collaboration.
Consistency is an important aspect of displaying authors in data science projects because it ensures that the information is clear, concise, and easy to understand. Inconsistent formatting can lead to confusion and make it difficult for readers to find and cite the work.
- Clarity: Consistent formatting helps to improve the clarity of the author information. When the authors’ names and affiliations are displayed in the same format throughout the project, it is easier for readers to quickly identify the authors and their institutional affiliations.
- Conciseness: Consistent formatting also helps to improve the conciseness of the author information. When the authors’ names and affiliations are displayed in a consistent format, it reduces the amount of space required to display the information.
- Ease of Use: Consistent formatting makes it easier for readers to find and use the author information. When the authors’ names and affiliations are displayed in the same format throughout the project, it is easier for readers to locate the information they need.
- Professionalism: Consistent formatting gives the project a more professional appearance. When the authors’ names and affiliations are displayed in a consistent format, it shows that the author has taken care to ensure that the project is well-presented.
In conclusion, consistency is an important aspect of displaying authors in data science projects. By using the same format for displaying the authors’ names and affiliations throughout the project, you can help to ensure that the information is clear, concise, easy to understand, and professional.
In the context of displaying authors in data science projects, prominence refers to the visibility and importance given to the authors’ names and affiliations within the project. By prominently displaying the authors’ information, you can ensure that they receive the recognition they deserve for their contributions to the project and that their work is easily discoverable by others.
- Clarity and Accessibility: Prominent display of authors’ names and affiliations enhances the clarity and accessibility of the project. When the authors’ information is easily visible, readers can quickly identify the individuals responsible for the work and learn more about their backgrounds and expertise. This transparency fosters trust and credibility for the project.
- Recognition and Attribution: Giving prominence to the authors’ names and affiliations ensures that they receive proper recognition and attribution for their contributions. This is particularly important in collaborative projects where multiple individuals may have played significant roles. By prominently displaying the authors’ information, you acknowledge their efforts and contributions to the project’s success.
- Discoverability and Collaboration: Prominent display of authors’ names and affiliations promotes the discoverability of the project and facilitates collaboration. When the authors’ information is easily accessible, other researchers and practitioners can easily identify and contact the authors to learn more about the project, explore potential collaborations, or build upon the work.
In conclusion, prominently displaying the authors’ names and affiliations in data science projects is crucial for recognizing the authors’ contributions, enhancing the project’s clarity and accessibility, and promoting discoverability and collaboration. By giving due prominence to the authors’ information, you can contribute to a culture of transparency, attribution, and knowledge sharing within the data science community.
Transparency is a crucial component of displaying authors in data science projects. By being transparent about the process used to identify and display the authors, you can ensure that the information is accurate, complete, and unbiased. This is important for several reasons:
- Accuracy: Transparency helps to ensure that the authors’ names and affiliations are displayed correctly. This is important because incorrect information can lead to confusion and make it difficult for others to find and cite the work.
- Completeness: Transparency helps to ensure that all of the authors who contributed to the project are included. This is important because incomplete information can lead to some authors not receiving credit for their work.
- Unbiased: Transparency helps to ensure that the process used to identify and display the authors is unbiased. This is important because bias can lead to certain authors being excluded or given less prominence than they deserve.
There are several ways to be transparent about the process used to identify and display the authors. One common approach is to include a section in the project documentation that describes the process. This section should include information about how the authors were identified, how their contributions were evaluated, and how the decision was made to include or exclude certain authors.
Another approach to transparency is to use a transparent and well-documented process for identifying and displaying authors. This process should be made available to anyone who is interested in learning more about it. By following these guidelines, you can help to ensure that the authors of data science projects receive the credit they deserve for their work.
Transparency in displaying authors is important for building trust and credibility. When readers know that the process used to identify and display the authors is transparent, they are more likely to trust the information that is presented. This trust is essential for the dissemination and adoption of data science research.
Timeliness, in the context of displaying authors in data science projects, refers to the prompt acknowledgment and recognition of the authors’ contributions after the project’s completion. This aspect is crucial for several reasons:
- Preservation of Authorship Rights: Timely display of authors’ information helps safeguard their authorship rights. By promptly attributing the work to the rightful individuals, you prevent any potential disputes or claims of unrecognized contributions.
- Respect for Authors’ Contributions: Displaying authors’ names and affiliations swiftly demonstrates respect for their efforts and acknowledges the value of their contributions. This recognition motivates authors to continue contributing to the field and fosters a sense of appreciation within the research community.
- Facilitating Collaboration: Timely display of authors’ information enables other researchers and practitioners to promptly identify and connect with the authors. This facilitates collaboration, knowledge exchange, and the building of a collaborative research network.
- Project Visibility and Dissemination: Prompt display of authors’ affiliations enhances the visibility and dissemination of the project’s findings. By making the authors’ names and affiliations known, it becomes easier for the work to reach a wider audience and gain recognition within the scientific community.
In summary, timeliness in displaying authors’ names and affiliations in data science projects is essential for preserving authorship rights, respecting authors’ contributions, facilitating collaboration, enhancing project visibility, and promoting the dissemination of research findings.
Sustainability, in the context of displaying authors in data science projects, refers to the ability to maintain the accuracy, completeness, and accessibility of the authors’ information over time. This is important because data science projects can be updated, revised, or extended over time, and it is essential that the authors’ information remains current and accessible.
- Data Management: One aspect of sustainability is data management. It is important to store the authors’ information in a structured and organized manner, using a system that is designed to preserve the integrity of the data over time. This may involve using a database, a version control system, or a dedicated metadata repository.
- Data Integrity: Another aspect of sustainability is data integrity. It is important to ensure that the authors’ information is accurate and complete, and that it is not subject to unauthorized changes or deletions. This may involve implementing data validation procedures, using checksums or other data integrity checks, and regularly backing up the data.
- Data Accessibility: The final aspect of sustainability is data accessibility. It is important to make the authors’ information easily accessible to anyone who is interested in learning more about the project. This may involve publishing the authors’ information on a website, in a journal article, or in a conference proceeding.
By following these guidelines, you can help to ensure that the authors’ names and affiliations are displayed in a way that is sustainable over time. This will help to ensure that the authors receive the credit they deserve for their work, and that the project remains accessible to future researchers.
Displaying Authors in Data Science Projects
In the realm of data science, acknowledging the contributions of authors is a fundamental aspect of research integrity and scholarly communication. Displaying authors in data science projects involves properly attributing and recognizing the individuals responsible for the conception, execution, and dissemination of research findings.
The significance of displaying authors extends beyond mere recognition. It ensures that authors receive due credit for their intellectual contributions, fostering a culture of accountability and transparency within the scientific community. Proper attribution also enables readers to identify the expertise and perspectives behind the research, aiding in the evaluation and interpretation of findings.
Historically, the display of authors in scientific publications has undergone significant evolution. In the early days of scientific inquiry, authors were often listed in alphabetical order, regardless of their level of contribution. However, as the complexity and collaborative nature of research increased, the need for a more nuanced approach to author attribution became apparent.
Today, various guidelines and conventions have been established to guide the display of authors in data science projects. These guidelines typically involve specifying the order of authors based on their relative contributions, with the corresponding affiliations and roles clearly indicated. By adhering to these conventions, researchers ensure that the recognition and credit for authorship are fairly and accurately assigned.
In conclusion, displaying authors in data science projects is not merely a formality but an essential component of research integrity and scholarly communication. It recognizes the contributions of individuals, promotes transparency, and facilitates the evaluation and dissemination of research findings. As the field of data science continues to evolve, the responsible and ethical display of authors will remain a cornerstone of scientific practice.
FAQs on Displaying Authors in Data Science Projects
This section addresses frequently asked questions (FAQs) on the topic of displaying authors in data science projects. These FAQs aim to provide clear and concise answers to common concerns or misconceptions, ensuring a thorough understanding of the best practices and ethical considerations surrounding author attribution.
Question 1: Why is it important to display authors in data science projects?
Displaying authors in data science projects is crucial for several reasons. First and foremost, it acknowledges and gives due credit to the individuals who have contributed to the research and development of the project. Proper attribution fosters a culture of accountability and transparency within the scientific community.
Question 2: How should authors be ordered in a publication?
The order of authors in a publication typically reflects their relative contributions to the project. The most common approach is to list authors in descending order of their contributions, with the corresponding affiliations and roles clearly indicated.
Question 3: What are some ethical considerations related to author display?
Ethical considerations in author display include ensuring that all authors who have made significant contributions to the project are included, avoiding ghost or guest authorship, and accurately representing the roles and responsibilities of each author.
Question 4: How can I determine the appropriate level of contribution for authorship?
Determining the appropriate level of contribution for authorship can be subjective. However, some common criteria include substantial intellectual input, significant involvement in data collection or analysis, and drafting or revising the manuscript.
Question 5: What are the consequences of inaccurate or incomplete author display?
Inaccurate or incomplete author display can undermine the credibility of the research and lead to disputes over authorship rights. It can also hinder the recognition and advancement of individual researchers.
Question 6: How can I ensure that author display is accurate and ethical?
To ensure accurate and ethical author display, it is essential to establish clear authorship guidelines, involve all authors in the discussion and decision-making process, and maintain transparent communication throughout the project.
In summary, displaying authors in data science projects is a fundamental aspect of research integrity and scholarly communication. By adhering to ethical guidelines and best practices, researchers can ensure that authors are fairly and accurately recognized for their contributions, promoting transparency, accountability, and the dissemination of high-quality research.
Transitioning to the next article section:
This concludes the FAQs on displaying authors in data science projects. For further insights and guidance, please refer to the additional resources and references provided in the following section.
Conclusion
In conclusion, displaying authors in data science projects is a fundamental aspect of research integrity and scholarly communication. By adhering to ethical guidelines and best practices, researchers can ensure that authors are fairly and accurately recognized for their contributions, promoting transparency, accountability, and the dissemination of high-quality research.
Proper author display not only acknowledges the contributions of individuals but also fosters a culture of collaboration, trust, and recognition within the scientific community. It enables readers to identify the expertise and perspectives behind the research, aiding in the evaluation and interpretation of findings. As the field of data science continues to evolve, the responsible and ethical display of authors will remain a cornerstone of scientific practice.
Youtube Video:
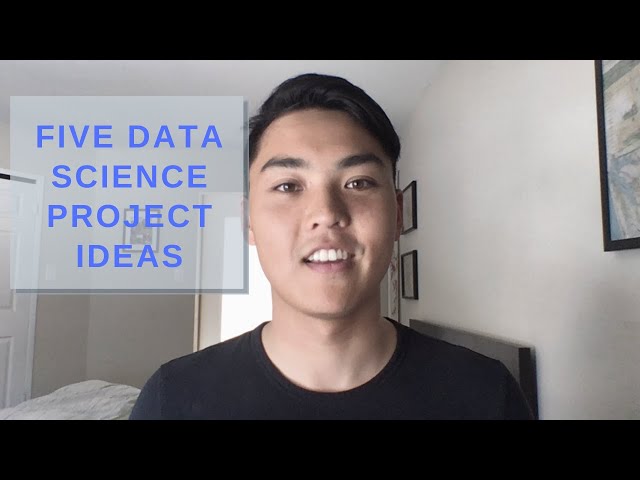