Data Science Projects Per Industry
Data science is a rapidly growing field that is having a major impact on a wide range of industries. By using data to solve problems and make better decisions, businesses can improve their efficiency, productivity, and profitability.There are many different types of data science projects that can be undertaken, and the specific type of project that is right for a particular business will depend on its specific needs and goals. However, some of the most common types of data science projects include:
Predictive analytics: This type of project uses data to predict future events. For example, a business might use predictive analytics to predict customer churn or demand for a new product.Prescriptive analytics: This type of project uses data to recommend actions that businesses can take to improve their performance. For example, a business might use prescriptive analytics to recommend changes to its marketing strategy or product development process.Data visualization: This type of project uses data to create visual representations that make it easier to understand and communicate data. For example, a business might use data visualization to create a dashboard that tracks key performance indicators or a map that shows the location of customers.
The benefits of data science projects can be significant. By using data to solve problems and make better decisions, businesses can:
Increase revenue: Data science projects can help businesses increase revenue by identifying new opportunities, improving marketing campaigns, and optimizing pricing.Reduce costs: Data science projects can help businesses reduce costs by identifying inefficiencies, improving supply chain management, and reducing fraud.Improve customer satisfaction: Data science projects can help businesses improve customer satisfaction by identifying customer needs, personalizing marketing messages, and resolving customer issues more quickly.
If you are considering undertaking a data science project, there are a few steps you should follow to ensure success:
Define your goals and objectives: What do you want to achieve with your data science project? What are the specific business problems you are trying to solve?Gather data: The quality of your data will have a major impact on the success of your data science project. Make sure you have access to the right data and that it is clean and accurate.Build a model: The model is the heart of your data science project. It is the algorithm that will use your data to make predictions or recommendations.Evaluate your results: Once you have built your model, you need to evaluate its performance to make sure it is accurate and reliable.Deploy your model: Once you are satisfied with the performance of your model, you need to deploy it so that it can be used to make decisions.
Data science projects can be a powerful tool for businesses of all sizes. By following these steps, you can ensure that your data science project is successful and delivers the results you are looking for.
Essential Aspects of Data Science Projects Per Industry
Data science projects are becoming increasingly important for businesses of all sizes. By using data to solve problems and make better decisions, businesses can improve their efficiency, productivity, and profitability. However, there are a number of key aspects that businesses need to consider when undertaking data science projects.
- Goals and objectives: What do you want to achieve with your data science project? What are the specific business problems you are trying to solve?
- Data: The quality of your data will have a major impact on the success of your data science project. Make sure you have access to the right data and that it is clean and accurate.
- Model: The model is the heart of your data science project. It is the algorithm that will use your data to make predictions or recommendations.
- Evaluation: Once you have built your model, you need to evaluate its performance to make sure it is accurate and reliable.
- Deployment: Once you are satisfied with the performance of your model, you need to deploy it so that it can be used to make decisions.
- Impact: What is the potential impact of your data science project? How will it improve your business?
By considering these key aspects, businesses can ensure that their data science projects are successful and deliver the results they are looking for. For example, a retail business might use data science to predict customer churn. By understanding the factors that contribute to customer churn, the business can develop targeted marketing campaigns to reduce churn and improve customer retention. Another example is a manufacturing business that might use data science to optimize its supply chain. By analyzing data on inventory levels, demand, and shipping costs, the business can identify inefficiencies and make changes to improve the efficiency of its supply chain.
Goals and objectives
Clearly defining the goals and objectives of a data science project is crucial for its success. This involves identifying the specific business problems that the project aims to solve and the desired outcomes. In the context of “data science projects per industry,” understanding the unique challenges and opportunities within each industry is essential for tailoring the project’s goals and objectives accordingly.
- Identifying Industry-Specific Problems: Different industries face distinct challenges that can be addressed through data science. For example, in the healthcare industry, a project might aim to improve patient outcomes by predicting the risk of certain diseases or personalizing treatment plans. In contrast, in the financial industry, a project might focus on detecting fraudulent transactions or optimizing investment strategies.
- Aligning with Business Objectives: The goals of a data science project should align with the overall objectives of the business. This ensures that the project contributes to the organization’s strategic priorities. For instance, a project aimed at increasing customer satisfaction in the retail industry might align with the business objective of enhancing customer loyalty and driving sales.
- Measuring Success: Defining clear metrics to measure the success of a data science project is essential. These metrics should be aligned with the project’s goals and objectives. For example, if the goal is to improve customer churn, the project’s success might be measured by a reduction in churn rate.
- Prioritizing Projects: With limited resources, organizations often need to prioritize data science projects based on their potential impact and alignment with business objectives. A well-defined set of goals and objectives helps in making informed decisions about which projects to undertake.
By carefully considering the goals and objectives of a data science project in the context of the specific industry, organizations can ensure that their projects are focused, relevant, and have a high probability of delivering valuable outcomes.
Data
In the context of “data science projects per industry,” the quality of data is paramount. Different industries generate and collect vast amounts of data, and the success of data science projects hinges on leveraging the right data and ensuring its accuracy and reliability.
- Data Relevance and Industry Specificity: The data used in data science projects should be relevant to the specific industry and aligned with the project’s objectives. For instance, in healthcare, utilizing patient health records, medical imaging data, and electronic health records is crucial. In contrast, in finance, data on financial transactions, market trends, and economic indicators is essential.
- Data Accuracy and Consistency: Data accuracy is vital to ensure that the insights and predictions generated from data science projects are reliable. Inaccurate or inconsistent data can lead to misleading results and incorrect decision-making. Data cleaning and preprocessing techniques are necessary to address missing values, outliers, and data inconsistencies.
- Data Completeness and Coverage: The completeness and coverage of data impact the robustness and generalizability of data science models. Sufficient data volume and representation of different scenarios are crucial to avoid biases and ensure that the models can handle real-world variations.
- Data Security and Privacy: Data security and privacy concerns are particularly relevant in industries dealing with sensitive information. Data science projects must comply with industry regulations and ethical guidelines to protect sensitive data and maintain confidentiality.
By paying meticulous attention to data quality, organizations can derive meaningful insights and make informed decisions through data science projects that drive innovation, improve efficiency, and enhance customer experiences within their respective industries.
Model
In the context of “data science projects per industry,” the model plays a pivotal role. Different industries have unique characteristics, challenges, and opportunities, and the choice of model should align with these specificities. Let’s explore some key facets of models in the context of data science projects per industry:
- Industry-Specific Algorithms: Industries often have their own set of specialized algorithms and techniques tailored to their unique data and problems. For instance, in healthcare, disease prediction models leverage medical knowledge and statistical methods, while in finance, algorithmic trading models incorporate market data and financial theories.
- Interpretability and Explainability: In industries where regulatory compliance or understanding the decision-making process is crucial, interpretable and explainable models are essential. These models provide insights into how predictions or recommendations are made, ensuring transparency and trust.
- Scalability and Efficiency: Industries dealing with massive datasets or real-time decision-making require scalable and efficient models. These models can handle large volumes of data, perform complex computations, and deliver timely results, enabling organizations to keep pace with the ever-increasing data demands.
- Data Privacy and Security: Industries handling sensitive data must prioritize data privacy and security in their models. Privacy-preserving techniques and secure algorithms ensure that sensitive information is protected while still allowing for valuable insights to be extracted.
By carefully considering the specific industry context and tailoring models accordingly, organizations can harness the full potential of data science projects, driving innovation and achieving their business goals.
Evaluation
In the context of “data science projects per industry,” evaluation is a critical step to ensure the robustness and effectiveness of the developed models. Different industries have unique evaluation criteria and metrics aligned with their specific goals and requirements.
- Industry-Specific Evaluation Metrics: Evaluation metrics should be tailored to the specific industry context. For instance, in healthcare, models predicting disease risk may be evaluated based on metrics like sensitivity, specificity, and predictive value. In finance, models assessing credit risk might be evaluated using metrics like accuracy, precision, and recall.
- Real-World Performance: Evaluating models in a real-world setting is crucial. This involves deploying the model in a production environment and monitoring its performance over time. Real-world evaluation helps identify potential issues, biases, or limitations that may not be apparent during the development and testing phases.
- Data Drift and Model Maintenance: Over time, data patterns and distributions can change, leading to model degradation. Regular evaluation helps detect data drift and trigger necessary model maintenance, retraining, or adjustments to ensure ongoing accuracy and reliability.
- Interpretability and Explainability: In industries where regulatory compliance or understanding the decision-making process is critical, interpretable and explainable models are preferred. Evaluation should assess the model’s ability to provide clear and understandable explanations for its predictions or recommendations.
By carefully evaluating models in the context of each industry’s unique characteristics and requirements, organizations can ensure that their data science projects deliver accurate, reliable, and actionable insights that drive informed decision-making and business success.
Deployment
Deployment is a critical phase in the data science project lifecycle, especially in the context of “data science projects per industry.” It involves making the developed model accessible and operational within the industry-specific environment to generate actionable insights and drive decision-making.
Importance of Deployment in Data Science Projects per Industry
- Real-World Impact: Deployment enables the model to be used in real-world scenarios, where it can directly impact business operations and decision-making. This allows organizations to leverage data science insights to improve efficiency, optimize processes, and drive growth.
- Continuous Improvement: Deployment facilitates ongoing monitoring and evaluation of the model’s performance in a production environment. This enables data scientists to identify potential issues, gather feedback, and make necessary adjustments to enhance the model’s accuracy and effectiveness over time.
- Industry-Specific Integration: Deployment involves integrating the model with industry-specific systems and applications. This ensures that the model can seamlessly access relevant data, generate insights, and deliver actionable recommendations within the context of the industry’s unique requirements.
Examples of Deployment in Data Science Projects per Industry
- Healthcare: Deploying a predictive model to assist in disease diagnosis, treatment selection, or personalized medicine.
- Finance: Deploying a fraud detection model to identify and prevent fraudulent transactions in real-time.
- Manufacturing: Deploying a predictive maintenance model to optimize equipment performance and prevent costly breakdowns.
Practical Significance
Understanding the importance of deployment in data science projects per industry empowers organizations to:
- Maximize the value of their data science investments by translating models into tangible business outcomes.
- Gain a competitive edge by leveraging data-driven insights to make informed decisions and stay ahead in the industry.
- Foster a culture of data-driven decision-making, where data science is seamlessly integrated into business operations.
In conclusion, deployment is an essential component of data science projects per industry. By deploying models, organizations can harness the power of data science to solve industry-specific challenges, improve decision-making, and drive innovation.
Impact
In the context of “data science projects per industry,” understanding the potential impact of a project is paramount. The industry-specific nature of data science projects demands careful consideration of how the project will address industry-specific challenges and drive business improvement.
The impact of a data science project can manifest in various ways, such as:
- Revenue uplift: Data science projects can help businesses increase revenue by identifying new opportunities, optimizing pricing, or personalizing marketing campaigns.
- Cost reduction: By optimizing processes, improving supply chain management, or reducing fraud, data science projects can lead to significant cost savings.
- Improved customer satisfaction: Data science projects can enhance customer satisfaction by identifying customer needs, personalizing products or services, and resolving issues more efficiently.
- Increased efficiency and productivity: Automating tasks, optimizing workflows, and providing data-driven insights can boost efficiency and productivity across industries.
- Competitive advantage: Data science projects can provide businesses with a competitive edge by enabling them to make better decisions, innovate faster, and stay ahead of the curve.
Consider the example of a retail company using data science to predict customer churn. By analyzing customer data, the company can identify factors contributing to churn and develop targeted interventions to retain valuable customers. This project’s impact could translate into increased customer loyalty, reduced churn rate, and ultimately higher revenue.
In conclusion, understanding the potential impact of data science projects per industry is crucial for businesses to justify investments, set realistic expectations, and align projects with strategic objectives. By thoroughly assessing the impact, organizations can make informed decisions and maximize the value derived from their data science initiatives.
In today’s data-driven business landscape, organizations across industries are leveraging data science to gain valuable insights, optimize operations, and drive innovation. Data science projects per industry involve the application of data science techniques and technologies to address specific challenges and opportunities within different industry sectors.
Data science projects per industry offer numerous benefits, including:
- Improved decision-making: Data science provides businesses with data-driven insights to make informed decisions, reducing the reliance on guesswork and intuition.
- Increased efficiency: Data science can automate tasks, optimize processes, and improve supply chain management, leading to increased efficiency and cost savings.
- Enhanced customer experience: Data science helps businesses understand their customers’ needs and preferences, enabling them to deliver personalized products, services, and experiences.
- Competitive advantage: By leveraging data science, businesses can gain a competitive edge by identifying new opportunities, developing innovative products, and staying ahead of industry trends.
Examples of data science projects per industry include:
- Predictive maintenance in manufacturing: Using data to predict when equipment is likely to fail, enabling proactive maintenance and reducing downtime.
- Fraud detection in financial services: Analyzing transaction data to identify and prevent fraudulent activities, protecting customers and the organization.
- Personalized marketing in retail: Leveraging customer data to create targeted marketing campaigns, increasing customer engagement and conversion rates.
- Disease prediction in healthcare: Using data to identify individuals at risk of developing certain diseases, enabling early intervention and preventive measures.
Organizations undertaking data science projects per industry should consider factors such as data availability, industry-specific regulations, and the availability of skilled data scientists. By carefully planning and executing data science projects, businesses can harness the power of data to drive growth, improve customer satisfaction, and gain a competitive advantage.
FAQs on Data Science Projects Per Industry
Question 1: What are the key benefits of undertaking data science projects per industry?
Data science projects per industry offer numerous benefits, including improved decision-making, increased efficiency, enhanced customer experience, and competitive advantage.
Question 2: Can you provide some examples of data science projects per industry?
Examples include predictive maintenance in manufacturing, fraud detection in financial services, personalized marketing in retail, and disease prediction in healthcare.
Question 3: What factors should businesses consider before undertaking data science projects per industry?
Organizations should consider data availability, industry-specific regulations, and the availability of skilled data scientists.
Question 4: How can businesses ensure the success of data science projects per industry?
Careful planning, execution, and alignment with industry-specific needs are crucial for successful data science projects.
Question 5: What are the challenges associated with data science projects per industry?
Challenges may include data quality and availability, the need for specialized expertise, and the integration of data science solutions into existing systems.
Question 6: How can organizations overcome the challenges associated with data science projects per industry?
Overcoming challenges requires a collaborative approach, investment in data infrastructure, and the development of industry-specific expertise.
Summary: Data science projects per industry offer significant benefits, but careful consideration of industry-specific factors and challenges is essential for success.
Conclusion on Data Science Projects Per Industry
Data science projects per industry have emerged as a powerful tool for organizations to address industry-specific challenges, optimize operations, and drive innovation. By leveraging data science techniques and technologies, businesses can gain valuable insights, improve decision-making, and gain a competitive advantage.
As the volume and variety of data continue to grow, data science projects per industry will become increasingly important. Organizations that embrace data science and invest in building their data science capabilities will be well-positioned to succeed in the digital age. Thoughtful consideration of industry-specific factors and challenges is crucial for the successful execution of data science projects. Collaboration, investment in data infrastructure, and the development of industry-specific expertise are key to unlocking the full potential of data science.
Youtube Video:
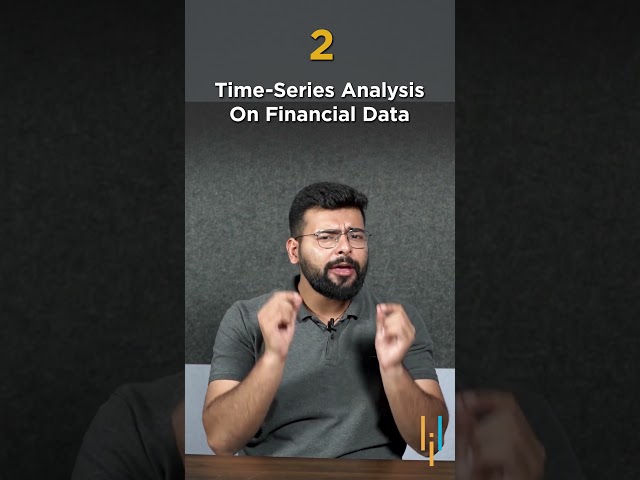